Integration of image and displacement monitoring techniques for masonry infrastructure
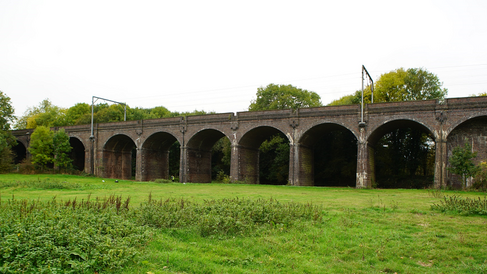
Principal Investigator: Dr Matt DeJong
Currently, the condition of masonry arch bridges are predominantly assessed via manual visual inspection. This process carries risk and cost due to the need for an inspection engineer to access sites in the proximity of busy railway lines and roads. Manual visual inspection is also known to be subjective, relying on the inspection engineer’s interpretation of the structures condition.
The collection of image and laser scan data is becoming increasingly fast (and this will continue with the use of drones for this purpose). There is therefore a large opportunity to collect and use this data to automate the visual inspection process through digital means. This project looks to identify and classify defects on the surface of masonry arch bridges from image data, and to locate these on a three-dimensional model of the structure using geometry data determined from laser scanning. This damage model of the structure will be used to inform its condition assessment.
The focus of the work in the past year has been on automated detection of defects in images of masonry. Masonry surfaces are often rough, and the grout-line patterns create a non-homogenous surface, making defect detection harder. Therefore, as a precursor to defect detection, a methodology has been developed to filter the individual bricks out from masonry images, enabling defect detection on the more homogeneous brick surface. This uses a deterministic approach, first detecting the position of grout-lines, and from these positions determining the brick spacing pattern, to then remove false grout-lines and add undetected grout-lines. The methodology has then been tested by applying a rudimentary crack detection process on masonry images with the grout lines masked. This applies a simple edge detection procedure and uses the geometry of detected points relative to one another to filter out genuine cracks from background noise.
Moving forward, the work will involve initially identifying the types of defects that are important to record on the structure and the ways in which these defects have an impact on the condition of the structure. Image and laser scan data from many different masonry arch bridges around the Cambridgeshire area will also be recorded to capture examples of the various defect types. This data will then be used to train and test a machine learning algorithm to identify and classify defects.